DOE Chooses Alumnus Wild for Early Career Research Award
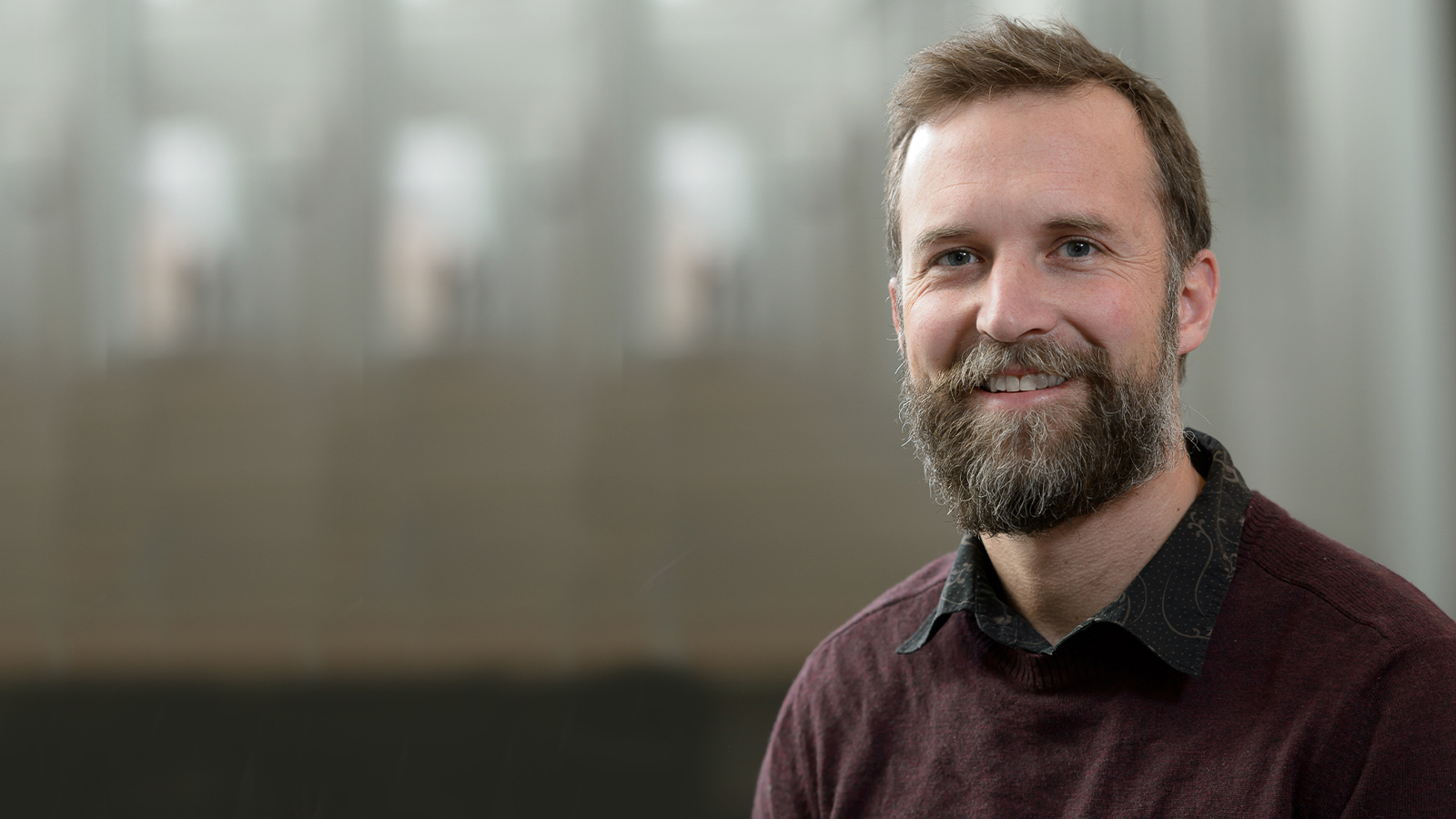
A project designed to improve the efficiency of scientific machine learning for high-performance computing (HPC) has earned an Early Career Research award for a Department of Energy Computational Science Graduate Fellowship (DOE CSGF) alumnus.
Stefan Wild, a computational mathematician in Argonne National Laboratory’s Laboratory for Applied Mathematics, Numerical Software and Statistics, will receive at least $500,000 per year for five years to support his work. The DOE Office of Advanced Scientific Computing Research selected the project. Wild was a fellow from 2005 to 2008.

Wild’s project, “Structure-Exploiting, Adaptive, Zeroth-Order Optimization to Improve Efficiency,” will address complex design, decision and control problems. Mathematical optimization problems – calculations that seek to find the best solution from all possibilities, such as the shortest, fastest route to multiple destinations – often require derivative information related to the rate of change in a quantity. Those requirements hamper their solution. Wild plans to develop mathematical methods that help scientists train and calibrate models, reduce risk and design improved experiments even when the requirements can’t be met, an Argonne release says.
The research will establish a robust mathematical foundation for scientific machine learning and optimization in increasingly complex HPC environments.
Wild earned bachelor’s and master’s degrees in applied mathematics at the University of Colorado Boulder and master’s and doctoral degrees in operations research at Cornell University.
Image caption: Stefan Wild’s mathematical algorithms explore complex design and decision spaces that depend on data from high-performance computing simulations and experimental and observational data. Credit: Stefan Wild, Argonne National Laboratory.