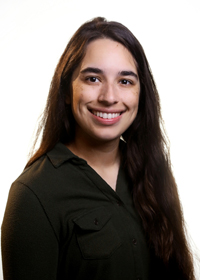
Sarah Greer
Massachusetts Institute of Technology
Sarah Greer comes from a globe-trotting family that followed her geophysicist father’s oil and gas industry career. He met Greer’s mother, a native of Burma (now Myanmar), while working there. Greer was born in Louisiana but the family moved to Egypt when she was an infant. Five years later they were in Norway and after three years they moved to the Houston area, where Greer finished her schooling.
Following her father’s interests, Greer entered the University of Texas at Austin to study geology and she discovered computational science’s capacity to elucidate subsurface structures. She added a math major and did research with Sergey Fomel, who “set me on the path of looking at things from a computational perspective for geophysics problems,” Greer says.
Her studies then – and now – revolve around seismic images. Surface-based sensors produce these pictures by recording sound waves that emanate from a source, such as an explosion or ground-shaking truck, and then reflect and refract off underground rock and soil layers. The information can help identify oil and gas deposits, geothermal energy sources, or structures for sequestering carbon dioxide from power plants.
Greer’s undergraduate research, more data processing than imaging, was similar to her father’s pursuits. But the Department of Energy Computational Science Graduate Fellowship (DOE CSGF) recipient has changed course since beginning doctoral studies at the Massachusetts Institute of Technology.
“I went from working for a geophysics advisor to a mathematician advisor (Laurent Demanet) for grad school. So I’m looking at things from more of a math perspective,” Greer says. Rather than center on physics and geology, her projects develop and apply analytic methods, research that’s “in between math and geophysics and computational science.”
Seismic imaging is an inverse process, starting with recorded data and working backwards to generate images of the subsurface structures that produced those data. The complexity and the mountain of seismic data complicate the task, which often requires high-performance computing.
Seismic images are hazy and noisy and poorly resolve subsurface structures. Greer works on superresolution, analytical methods to improve images to more closely match the features that produced them. “There are a few tricks that can be done on the math side so that the resolution can be higher when you take into account aspects of the waves that come back to the surface,” she says. That includes exploring full waveform inversion, which seeks the optimal solution while avoiding false answers as the algorithm searches parameters.

For her 2019 Los Alamos National Laboratory practicum, Greer worked with Daniel O’Malley on a similar inverse problem: characterizing subsurface fluid flow. The project also tested using quantum computers for practical problems. These machines use physics that govern matter’s tiniest scales to boost computational power, but they face many technical obstacles.
Greer used Los Alamos’s D-Wave 2000Q quantum annealer, a quantum machine tailored for optimization problems, which seek the best or lowest-energy solution from among myriad options. Using synthetic surface measurements of subsurface water pressure over a specified area, Greer and O’Malley developed a model that iteratively updated calculations to best estimate the permeability of underground soil and rock.
The D-Wave computer’s capacity was still limited, so the researchers restricted the problem size and constrained possible measurements to one of two values.
Greer later used the technique for a seismic inversion problem similar to her thesis research. Using synthetic seismic measurements over a chosen land area, she and O’Malley calculated underground pressure wave velocity. As in the hydrological study, the model was constrained to one of two values at each point.
Greer dove into machine learning (ML) for inversion on a 2020 (virtual) Los Alamos practicum. She worked with Youzuo Lin’s InversionNet, which trains a neural network on existing seismic wave-propagation data to predict values from new, previously unseen information. The problem: Many ML techniques can’t adapt to unexpected or unexplained data on which they haven’t trained, such as statistical noise.
Greer worked to improve InversionNet’s ability to deal with unexpected data, adding an additional training parameter that relied on the underlying seismic physics. The resulting algorithm worked well but is so computationally demanding that using it may not be worth the improved results.
ML is widespread in Greer’s field, but she has mostly eschewed it to use classical methods. She worked with Lin to learn more about the technique. She’ll do another Los Alamos practicum in summer 2021 with O’Malley and Lin, aiming to combine aspects of their research interests.
The practicums have led Greer to consider working at Los Alamos or another DOE lab after graduation, projected for 2023.
Image caption: A velocity model of a single wave-scattering object (left), the resulting standard reverse-time migration (RTM) image (center), and the new RTM image using the zero-phase imaging condition (right). Standard imaging techniques may not completely resolve a single scatterer in the subsurface. This new imaging condition produces a superresolved image of the scatterer at the correct location in the subsurface. Credit: Sarah Greer.