Alumnus Leads Development of Machine-Learning Method for Materials
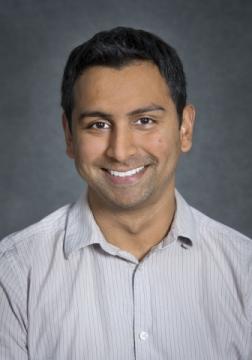
A team led by a Department of Energy Computational Science Graduate Fellowship (DOE CSGF) alumnus has shown that a machine-learning algorithm can find and predict new materials with useful properties based on an analysis of millions of scientific publications.
Anubhav Jain, a fellow from 2008-2011, led development of the algorithm in the Energy Storage & Distributed Resources Division at Lawrence Berkeley National Laboratory in California. The team trained a language-processing algorithm, called Word2vec, on 3.3 million abstracts from papers published in more than 1,000 journals between 1922 and 2018. By analyzing relationships between words, the algorithm was able to predict discoveries of new thermoelectric materials years in advance and suggest as-yet unknown substances that could have similar properties, a lab release said.
“Without telling it anything about materials science, it learned concepts like the periodic table and the crystal structure of metals,” Jain said. “That hinted at the potential of the technique. But probably the most interesting thing we figured out is you can use this algorithm to address gaps in materials research, things that people should study but haven’t studied so far.”
The research was published July 3 in the journal Nature. The lead author is Vahe Tshitoyan, a Berkeley Lab postdoctoral fellow now working at Google. The project could help accelerate the discovery and implementation of new, useful materials.
Jain earned a Ph.D. in materials science and engineering from the Massachusetts Institute of Technology in 2011.