An Enhanced Approach to Signal Analysis in the XENONnT Dark Matter Experiment
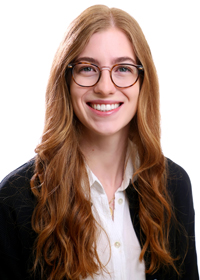
The XENONnT experiment is a direct detector searching for dark matter and neutrino signals, aided by extremely low background rates and purity. To reach the keV-scale threshold of detection necessary for sensitive searches, both physical backgrounds and artifacts in reconstruction must be rejected in a robust way. We developed a novel approach to signal classification and characterization in XENONnT using a Bayesian network model. The model can be used both to infer whether a detector signal is sourced from a scintillation or an ionization process and to reject unphysical background signals based solely on waveform shape. One advantage of our approach to signal characterization is an increased efficiency at the threshold of detection. In applying this method to electronic-recoil data taken during the first science run of XENONnT, we report a background-only fit to the data and precise detection of ultrarare nuclear decays.